注意
单击 此处 下载完整示例代码
PyTorch 数值套件教程¶
简介¶
量化在有效的情况下很有用,但在无法满足我们预期的精度时,很难确定问题所在。调试量化的精度问题并非易事,而且非常耗时。
调试的一个重要步骤是测量浮点模型及其对应的量化模型的统计数据,以了解它们在哪些方面差异最大。我们在 PyTorch 量化中构建了一套名为 PyTorch 数值套件的数值工具,用于测量量化模块和浮点模块之间的统计数据,以支持量化调试工作。即使对于具有良好精度的量化模型,PyTorch 数值套件仍然可以作为分析工具,帮助更好地理解模型中的量化误差,并为进一步优化提供指导。
PyTorch 数值套件目前支持通过静态量化和动态量化量化的模型,并提供统一的 API。
在本教程中,我们将首先使用 ResNet18 作为示例,演示如何在渴望模式下使用 PyTorch 数值套件测量静态量化模型和浮点模型之间的统计数据。然后,我们将使用基于 LSTM 的序列模型作为示例,演示 PyTorch 数值套件在动态量化模型中的用法。
用于静态量化的数值套件¶
设置¶
我们将从执行必要的导入开始
import numpy as np
import torch
import torch.nn as nn
import torchvision
from torchvision import models, datasets
import torchvision.transforms as transforms
import os
import torch.quantization
import torch.quantization._numeric_suite as ns
from torch.quantization import (
default_eval_fn,
default_qconfig,
quantize,
)
然后,我们加载预训练的浮点 ResNet18 模型,并将其量化到 qmodel 中。我们无法比较两个任意模型,只能比较浮点模型和源自它的量化模型。
float_model = torchvision.models.quantization.resnet18(weights=models.ResNet18_Weights.IMAGENET1K_V1, quantize=False)
float_model.to('cpu')
float_model.eval()
float_model.fuse_model()
float_model.qconfig = torch.quantization.default_qconfig
img_data = [(torch.rand(2, 3, 10, 10, dtype=torch.float), torch.randint(0, 1, (2,), dtype=torch.long)) for _ in range(2)]
qmodel = quantize(float_model, default_eval_fn, [img_data], inplace=False)
Downloading: "https://download.pytorch.org/models/resnet18-f37072fd.pth" to /var/lib/ci-user/.cache/torch/hub/checkpoints/resnet18-f37072fd.pth
0%| | 0.00/44.7M [00:00<?, ?B/s]
39%|###9 | 17.5M/44.7M [00:00<00:00, 183MB/s]
80%|#######9 | 35.6M/44.7M [00:00<00:00, 186MB/s]
100%|##########| 44.7M/44.7M [00:00<00:00, 185MB/s]
1. 比较浮点模型和量化模型的权重¶
我们通常想比较的第一件事是量化模型和浮点模型的权重。我们可以从 PyTorch 数值套件中调用 compare_weights()
来获取一个字典 wt_compare_dict
,其键对应于模块名称,每个条目都是一个字典,有两个键 ‘float’ 和 ‘quantized’,包含浮点权重和量化权重。 compare_weights()
接受浮点和量化状态字典,并返回一个字典,键对应于浮点权重,值是浮点权重和量化权重的字典
wt_compare_dict = ns.compare_weights(float_model.state_dict(), qmodel.state_dict())
print('keys of wt_compare_dict:')
print(wt_compare_dict.keys())
print("\nkeys of wt_compare_dict entry for conv1's weight:")
print(wt_compare_dict['conv1.weight'].keys())
print(wt_compare_dict['conv1.weight']['float'].shape)
print(wt_compare_dict['conv1.weight']['quantized'].shape)
keys of wt_compare_dict:
dict_keys(['conv1.weight', 'layer1.0.conv1.weight', 'layer1.0.conv2.weight', 'layer1.1.conv1.weight', 'layer1.1.conv2.weight', 'layer2.0.conv1.weight', 'layer2.0.conv2.weight', 'layer2.0.downsample.0.weight', 'layer2.1.conv1.weight', 'layer2.1.conv2.weight', 'layer3.0.conv1.weight', 'layer3.0.conv2.weight', 'layer3.0.downsample.0.weight', 'layer3.1.conv1.weight', 'layer3.1.conv2.weight', 'layer4.0.conv1.weight', 'layer4.0.conv2.weight', 'layer4.0.downsample.0.weight', 'layer4.1.conv1.weight', 'layer4.1.conv2.weight', 'fc._packed_params._packed_params'])
keys of wt_compare_dict entry for conv1's weight:
dict_keys(['float', 'quantized'])
torch.Size([64, 3, 7, 7])
torch.Size([64, 3, 7, 7])
一旦获得 wt_compare_dict
,用户就可以以任何他们想要的方式处理这个字典。这里举个例子,我们计算浮点模型和量化模型权重的量化误差,如下所示。计算量化张量 y
的信噪比 (SQNR)。SQNR 反映了最大标称信号强度与量化过程中引入的量化误差之间的关系。更高的 SQNR 对应于更低的量化误差。
def compute_error(x, y):
Ps = torch.norm(x)
Pn = torch.norm(x-y)
return 20*torch.log10(Ps/Pn)
for key in wt_compare_dict:
print(key, compute_error(wt_compare_dict[key]['float'], wt_compare_dict[key]['quantized'].dequantize()))
conv1.weight tensor(31.6638)
layer1.0.conv1.weight tensor(30.6450)
layer1.0.conv2.weight tensor(31.1528)
layer1.1.conv1.weight tensor(32.1438)
layer1.1.conv2.weight tensor(31.2477)
layer2.0.conv1.weight tensor(30.9890)
layer2.0.conv2.weight tensor(28.8233)
layer2.0.downsample.0.weight tensor(31.5558)
layer2.1.conv1.weight tensor(30.7668)
layer2.1.conv2.weight tensor(28.4516)
layer3.0.conv1.weight tensor(30.9247)
layer3.0.conv2.weight tensor(26.6841)
layer3.0.downsample.0.weight tensor(28.7825)
layer3.1.conv1.weight tensor(28.9707)
layer3.1.conv2.weight tensor(25.6784)
layer4.0.conv1.weight tensor(26.8495)
layer4.0.conv2.weight tensor(25.8394)
layer4.0.downsample.0.weight tensor(28.6355)
layer4.1.conv1.weight tensor(26.8758)
layer4.1.conv2.weight tensor(28.4319)
fc._packed_params._packed_params tensor(32.6505)
另一个例子是,wt_compare_dict
也可以用于绘制浮点模型和量化模型权重的直方图。
import matplotlib.pyplot as plt
f = wt_compare_dict['conv1.weight']['float'].flatten()
plt.hist(f, bins = 100)
plt.title("Floating point model weights of conv1")
plt.show()
q = wt_compare_dict['conv1.weight']['quantized'].flatten().dequantize()
plt.hist(q, bins = 100)
plt.title("Quantized model weights of conv1")
plt.show()
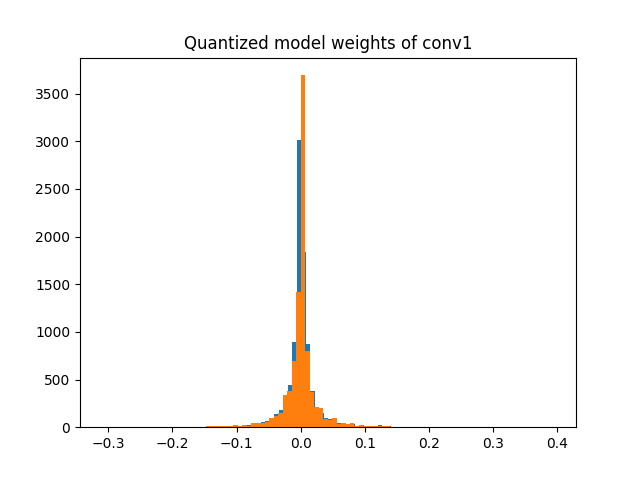
2. 在对应位置比较浮点模型和量化模型¶
第二个工具允许在对应位置比较浮点模型和量化模型在相同输入下的权重和激活,如下图所示。红色箭头指示比较的位置。
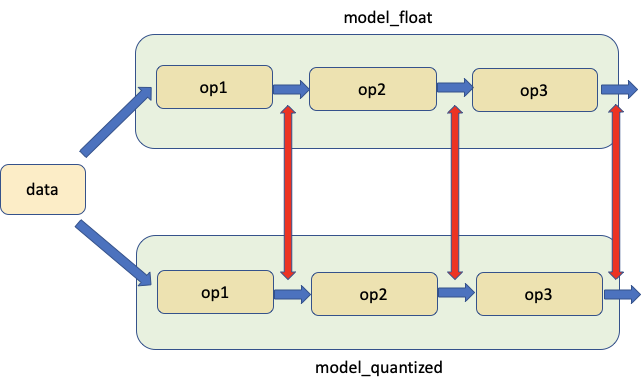
我们从 PyTorch 数值套件中调用 compare_model_outputs()
来获取在给定输入数据下浮点模型和量化模型在对应位置的激活。此 API 返回一个字典,模块名称作为键。每个条目本身都是一个字典,有两个键 ‘float’ 和 ‘quantized’,包含激活。
data = img_data[0][0]
# Take in floating point and quantized model as well as input data, and returns a dict, with keys
# corresponding to the quantized module names and each entry being a dictionary with two keys 'float' and
# 'quantized', containing the activations of floating point and quantized model at matching locations.
act_compare_dict = ns.compare_model_outputs(float_model, qmodel, data)
print('keys of act_compare_dict:')
print(act_compare_dict.keys())
print("\nkeys of act_compare_dict entry for conv1's output:")
print(act_compare_dict['conv1.stats'].keys())
print(act_compare_dict['conv1.stats']['float'][0].shape)
print(act_compare_dict['conv1.stats']['quantized'][0].shape)
keys of act_compare_dict:
dict_keys(['conv1.stats', 'layer1.0.conv1.stats', 'layer1.0.conv2.stats', 'layer1.0.add_relu.stats', 'layer1.1.conv1.stats', 'layer1.1.conv2.stats', 'layer1.1.add_relu.stats', 'layer2.0.conv1.stats', 'layer2.0.conv2.stats', 'layer2.0.downsample.0.stats', 'layer2.0.add_relu.stats', 'layer2.1.conv1.stats', 'layer2.1.conv2.stats', 'layer2.1.add_relu.stats', 'layer3.0.conv1.stats', 'layer3.0.conv2.stats', 'layer3.0.downsample.0.stats', 'layer3.0.add_relu.stats', 'layer3.1.conv1.stats', 'layer3.1.conv2.stats', 'layer3.1.add_relu.stats', 'layer4.0.conv1.stats', 'layer4.0.conv2.stats', 'layer4.0.downsample.0.stats', 'layer4.0.add_relu.stats', 'layer4.1.conv1.stats', 'layer4.1.conv2.stats', 'layer4.1.add_relu.stats', 'fc.stats', 'quant.stats'])
keys of act_compare_dict entry for conv1's output:
dict_keys(['float', 'quantized'])
torch.Size([2, 64, 5, 5])
torch.Size([2, 64, 5, 5])
这个字典可以用来比较和计算浮点模型和量化模型激活的量化误差,如下所示。
for key in act_compare_dict:
print(key, compute_error(act_compare_dict[key]['float'][0], act_compare_dict[key]['quantized'][0].dequantize()))
conv1.stats tensor(37.1388, grad_fn=<MulBackward0>)
layer1.0.conv1.stats tensor(30.1562, grad_fn=<MulBackward0>)
layer1.0.conv2.stats tensor(29.0511, grad_fn=<MulBackward0>)
layer1.0.add_relu.stats tensor(32.7605, grad_fn=<MulBackward0>)
layer1.1.conv1.stats tensor(30.1330, grad_fn=<MulBackward0>)
layer1.1.conv2.stats tensor(26.3872, grad_fn=<MulBackward0>)
layer1.1.add_relu.stats tensor(30.0649, grad_fn=<MulBackward0>)
layer2.0.conv1.stats tensor(26.9528, grad_fn=<MulBackward0>)
layer2.0.conv2.stats tensor(26.7812, grad_fn=<MulBackward0>)
layer2.0.downsample.0.stats tensor(23.2544, grad_fn=<MulBackward0>)
layer2.0.add_relu.stats tensor(26.2048, grad_fn=<MulBackward0>)
layer2.1.conv1.stats tensor(25.6735, grad_fn=<MulBackward0>)
layer2.1.conv2.stats tensor(24.6564, grad_fn=<MulBackward0>)
layer2.1.add_relu.stats tensor(26.0816, grad_fn=<MulBackward0>)
layer3.0.conv1.stats tensor(26.9846, grad_fn=<MulBackward0>)
layer3.0.conv2.stats tensor(26.8694, grad_fn=<MulBackward0>)
layer3.0.downsample.0.stats tensor(25.1453, grad_fn=<MulBackward0>)
layer3.0.add_relu.stats tensor(24.8748, grad_fn=<MulBackward0>)
layer3.1.conv1.stats tensor(31.0022, grad_fn=<MulBackward0>)
layer3.1.conv2.stats tensor(26.1478, grad_fn=<MulBackward0>)
layer3.1.add_relu.stats tensor(25.5775, grad_fn=<MulBackward0>)
layer4.0.conv1.stats tensor(27.4940, grad_fn=<MulBackward0>)
layer4.0.conv2.stats tensor(27.2149, grad_fn=<MulBackward0>)
layer4.0.downsample.0.stats tensor(22.5105, grad_fn=<MulBackward0>)
layer4.0.add_relu.stats tensor(21.2105, grad_fn=<MulBackward0>)
layer4.1.conv1.stats tensor(26.5055, grad_fn=<MulBackward0>)
layer4.1.conv2.stats tensor(18.5702, grad_fn=<MulBackward0>)
layer4.1.add_relu.stats tensor(18.5091, grad_fn=<MulBackward0>)
fc.stats tensor(20.7117, grad_fn=<MulBackward0>)
quant.stats tensor(47.9043)
如果我们想要对多个输入数据进行比较,可以执行以下操作。通过将记录器附加到浮点模块和量化模块(如果它们在 white_list
中),来准备模型。默认记录器是 OutputLogger
,默认 white_list 是 DEFAULT_NUMERIC_SUITE_COMPARE_MODEL_OUTPUT_WHITE_LIST
ns.prepare_model_outputs(float_model, qmodel)
for data in img_data:
float_model(data[0])
qmodel(data[0])
# Find the matching activation between floating point and quantized modules, and return a dict with key
# corresponding to quantized module names and each entry being a dictionary with two keys 'float'
# and 'quantized', containing the matching floating point and quantized activations logged by the logger
act_compare_dict = ns.get_matching_activations(float_model, qmodel)
上述 API 中使用的默认记录器是 OutputLogger
,用于记录模块的输出。我们可以继承自基础 Logger
类并创建我们自己的记录器来执行不同的功能。例如,我们可以创建一个新的 MyOutputLogger
类,如下所示。
class MyOutputLogger(ns.Logger):
r"""Customized logger class
"""
def __init__(self):
super(MyOutputLogger, self).__init__()
def forward(self, x):
# Custom functionalities
# ...
return x
然后我们可以将这个记录器传递到上面的 API 中,例如
data = img_data[0][0]
act_compare_dict = ns.compare_model_outputs(float_model, qmodel, data, logger_cls=MyOutputLogger)
或
ns.prepare_model_outputs(float_model, qmodel, MyOutputLogger)
for data in img_data:
float_model(data[0])
qmodel(data[0])
act_compare_dict = ns.get_matching_activations(float_model, qmodel)
3. 使用相同输入数据比较量化模型中的模块与其浮点等效模块¶
第三个工具允许比较模型中的量化模块与其浮点对应模块,对两者输入相同的数据并比较它们的输出,如下图所示。
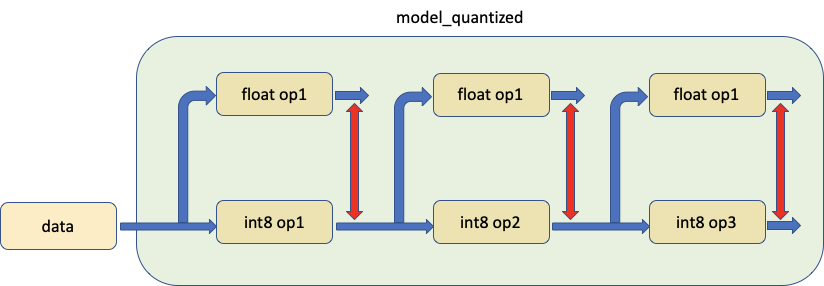
在实践中,我们调用 prepare_model_with_stubs() 来交换要与 Shadow 模块比较的量化模块,如下图所示
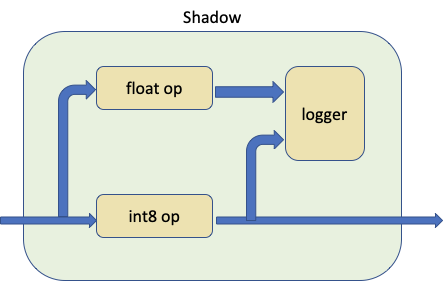
Shadow 模块将量化模块、浮点模块和记录器作为输入,并在内部创建一个前向路径,使浮点模块对量化模块进行阴影处理,共享相同的输入张量。
记录器可以自定义,默认记录器是 ShadowLogger
,它将保存量化模块和浮点模块的输出,这些输出可以用于计算模块级别的量化误差。
注意,在每次调用 compare_model_outputs()
和 compare_model_stub()
之前,我们需要有一个干净的浮点模型和量化模型。这是因为 compare_model_outputs()
和 compare_model_stub()
会就地修改浮点模型和量化模型,如果一个接一个地调用它们,就会导致意外的结果。
float_model = torchvision.models.quantization.resnet18(weights=models.ResNet18_Weights.IMAGENET1K_V1, quantize=False)
float_model.to('cpu')
float_model.eval()
float_model.fuse_model()
float_model.qconfig = torch.quantization.default_qconfig
img_data = [(torch.rand(2, 3, 10, 10, dtype=torch.float), torch.randint(0, 1, (2,), dtype=torch.long)) for _ in range(2)]
qmodel = quantize(float_model, default_eval_fn, [img_data], inplace=False)
在下面的例子中,我们从 PyTorch 数值套件中调用 compare_model_stub()
来比较 QuantizableBasicBlock
模块与其浮点等效模块。此 API 返回一个字典,键对应于模块名称,每个条目都是一个字典,有两个键 ‘float’ 和 ‘quantized’,包含量化模块及其匹配的浮点阴影模块的输出张量。
data = img_data[0][0]
module_swap_list = [torchvision.models.quantization.resnet.QuantizableBasicBlock]
# Takes in floating point and quantized model as well as input data, and returns a dict with key
# corresponding to module names and each entry being a dictionary with two keys 'float' and
# 'quantized', containing the output tensors of quantized module and its matching floating point shadow module.
ob_dict = ns.compare_model_stub(float_model, qmodel, module_swap_list, data)
print('keys of ob_dict:')
print(ob_dict.keys())
print("\nkeys of ob_dict entry for layer1.0's output:")
print(ob_dict['layer1.0.stats'].keys())
print(ob_dict['layer1.0.stats']['float'][0].shape)
print(ob_dict['layer1.0.stats']['quantized'][0].shape)
keys of ob_dict:
dict_keys(['layer1.0.stats', 'layer1.1.stats', 'layer2.0.stats', 'layer2.1.stats', 'layer3.0.stats', 'layer3.1.stats', 'layer4.0.stats', 'layer4.1.stats'])
keys of ob_dict entry for layer1.0's output:
dict_keys(['float', 'quantized'])
torch.Size([64, 3, 3])
torch.Size([64, 3, 3])
这个字典可以用来比较和计算模块级别的量化误差。
for key in ob_dict:
print(key, compute_error(ob_dict[key]['float'][0], ob_dict[key]['quantized'][0].dequantize()))
layer1.0.stats tensor(32.7203)
layer1.1.stats tensor(34.8070)
layer2.0.stats tensor(29.3657)
layer2.1.stats tensor(31.0864)
layer3.0.stats tensor(28.5980)
layer3.1.stats tensor(31.3857)
layer4.0.stats tensor(25.3010)
layer4.1.stats tensor(22.9801)
如果我们想要对多个输入数据进行比较,可以执行以下操作。
ns.prepare_model_with_stubs(float_model, qmodel, module_swap_list, ns.ShadowLogger)
for data in img_data:
qmodel(data[0])
ob_dict = ns.get_logger_dict(qmodel)
上述 API 中使用的默认记录器是 ShadowLogger
,用于记录量化模块及其匹配的浮点阴影模块的输出。我们可以继承自基础 Logger
类并创建我们自己的记录器来执行不同的功能。例如,我们可以创建一个新的 MyShadowLogger
类,如下所示。
class MyShadowLogger(ns.Logger):
r"""Customized logger class
"""
def __init__(self):
super(MyShadowLogger, self).__init__()
def forward(self, x, y):
# Custom functionalities
# ...
return x
然后我们可以将这个记录器传递到上面的 API 中,例如
data = img_data[0][0]
ob_dict = ns.compare_model_stub(float_model, qmodel, module_swap_list, data, logger_cls=MyShadowLogger)
或
ns.prepare_model_with_stubs(float_model, qmodel, module_swap_list, MyShadowLogger)
for data in img_data:
qmodel(data[0])
ob_dict = ns.get_logger_dict(qmodel)
动态量化的数值套件¶
数值套件 API 的设计方式使其适用于动态量化模型和静态量化模型。我们将使用一个同时包含 LSTM 和 Linear 模块的模型来演示数值套件在动态量化模型上的使用。这个模型与 LSTM 词语言模型动态量化教程中使用的模型相同 [1]。
设置¶
首先,我们定义模型如下。注意,在这个模型中,只有 nn.LSTM
和 nn.Linear
模块将被动态量化,而 nn.Embedding
在量化后将保持为浮点模块。
class LSTMModel(nn.Module):
"""Container module with an encoder, a recurrent module, and a decoder."""
def __init__(self, ntoken, ninp, nhid, nlayers, dropout=0.5):
super(LSTMModel, self).__init__()
self.encoder = nn.Embedding(ntoken, ninp)
self.rnn = nn.LSTM(ninp, nhid, nlayers, dropout=dropout)
self.decoder = nn.Linear(nhid, ntoken)
self.init_weights()
self.nhid = nhid
self.nlayers = nlayers
def init_weights(self):
initrange = 0.1
self.encoder.weight.data.uniform_(-initrange, initrange)
self.decoder.bias.data.zero_()
self.decoder.weight.data.uniform_(-initrange, initrange)
def forward(self, input, hidden):
emb = self.encoder(input)
output, hidden = self.rnn(emb, hidden)
decoded = self.decoder(output)
return decoded, hidden
def init_hidden(self, bsz):
weight = next(self.parameters())
return (weight.new_zeros(self.nlayers, bsz, self.nhid),
weight.new_zeros(self.nlayers, bsz, self.nhid))
然后我们创建 float_model
并将其量化成 qmodel。
ntokens = 10
float_model = LSTMModel(
ntoken = ntokens,
ninp = 512,
nhid = 256,
nlayers = 5,
)
float_model.eval()
qmodel = torch.quantization.quantize_dynamic(
float_model, {nn.LSTM, nn.Linear}, dtype=torch.qint8
)
1. 比较浮点模型和量化模型的权重¶
我们首先从 PyTorch 数值套件中调用 compare_weights()
来获取一个字典 wt_compare_dict
,其键对应于模块名称,每个条目都是一个字典,有两个键 ‘float’ 和 ‘quantized’,包含浮点权重和量化权重。
wt_compare_dict = ns.compare_weights(float_model.state_dict(), qmodel.state_dict())
一旦我们获得 wt_compare_dict
,就可以用来比较和计算浮点模型和量化模型权重的量化误差,如下所示。
for key in wt_compare_dict:
if wt_compare_dict[key]['quantized'].is_quantized:
print(key, compute_error(wt_compare_dict[key]['float'], wt_compare_dict[key]['quantized'].dequantize()))
else:
print(key, compute_error(wt_compare_dict[key]['float'], wt_compare_dict[key]['quantized']))
encoder.weight tensor(inf)
rnn._all_weight_values.0.param tensor(48.1323)
rnn._all_weight_values.1.param tensor(48.1355)
rnn._all_weight_values.2.param tensor(48.1213)
rnn._all_weight_values.3.param tensor(48.1506)
rnn._all_weight_values.4.param tensor(48.1348)
decoder._packed_params._packed_params tensor(48.0233)
上面 encoder.weight
条目中的 Inf 值是因为 encoder 模块没有被量化,并且权重在浮点模型和量化模型中是相同的。
2. 在对应位置比较浮点模型和量化模型¶
然后我们从 PyTorch 数值套件中调用 compare_model_outputs()
来获取在给定输入数据下浮点模型和量化模型在对应位置的激活。此 API 返回一个字典,模块名称作为键。每个条目本身都是一个字典,有两个键 ‘float’ 和 ‘quantized’,包含激活。注意,这个序列模型有两个输入,我们可以将两个输入都传递到 compare_model_outputs()
和 compare_model_stub()
中。
input_ = torch.randint(ntokens, (1, 1), dtype=torch.long)
hidden = float_model.init_hidden(1)
act_compare_dict = ns.compare_model_outputs(float_model, qmodel, input_, hidden)
print(act_compare_dict.keys())
dict_keys(['encoder.stats', 'rnn.stats', 'decoder.stats'])
这个字典可以用来比较和计算浮点模型和量化模型激活的量化误差,如下所示。这个模型中的 LSTM 模块有两个输出,在这个例子中,我们计算第一个输出的误差。
for key in act_compare_dict:
print(key, compute_error(act_compare_dict[key]['float'][0][0], act_compare_dict[key]['quantized'][0][0]))
encoder.stats tensor(inf, grad_fn=<MulBackward0>)
rnn.stats tensor(54.7745, grad_fn=<MulBackward0>)
decoder.stats tensor(37.2282, grad_fn=<MulBackward0>)
3. 使用相同输入数据比较量化模型中的模块与其浮点等效模块¶
接下来,我们从 PyTorch 数值套件中调用 compare_model_stub()
来比较 LSTM 和 Linear 模块与其浮点等效模块。此 API 返回一个字典,键对应于模块名称,每个条目都是一个字典,有两个键 ‘float’ 和 ‘quantized’,包含量化模块及其匹配的浮点阴影模块的输出张量。
我们首先重置模型。
float_model = LSTMModel(
ntoken = ntokens,
ninp = 512,
nhid = 256,
nlayers = 5,
)
float_model.eval()
qmodel = torch.quantization.quantize_dynamic(
float_model, {nn.LSTM, nn.Linear}, dtype=torch.qint8
)
接下来,我们从 PyTorch 数值套件中调用 compare_model_stub()
来比较 LSTM 和 Linear 模块与其浮点等效模块。此 API 返回一个字典,键对应于模块名称,每个条目都是一个字典,有两个键 ‘float’ 和 ‘quantized’,包含量化模块及其匹配的浮点阴影模块的输出张量。
dict_keys(['rnn.stats', 'decoder.stats'])
这个字典可以用来比较和计算模块级别的量化误差。
for key in ob_dict:
print(key, compute_error(ob_dict[key]['float'][0], ob_dict[key]['quantized'][0]))
rnn.stats tensor(54.6112)
decoder.stats tensor(40.2375)
40 dB 的 SQNR 较高,这种情况表明浮点模型和量化模型之间具有非常好的数值一致性。