注意
点击 此处 下载完整的示例代码
Python 自定义算子¶
如何将用 Python 编写的自定义算子与 PyTorch 集成
如何使用
torch.library.opcheck
测试自定义算子
PyTorch 2.4 或更高版本
PyTorch 提供了一个大型算子库,这些算子可以在张量上工作(例如 torch.add
、torch.sum
等)。但是,您可能希望在 PyTorch 中使用新的自定义算子,也许是由第三方库编写的。本教程展示了如何包装 Python 函数,使其行为类似于 PyTorch 原生算子。您可能希望在 PyTorch 中创建自定义算子的原因包括
将任意 Python 函数视为相对于
torch.compile
的不透明可调用对象(即,防止torch.compile
跟踪到该函数内部)。为任意 Python 函数添加训练支持
请注意,如果您的操作可以用现有的 PyTorch 算子的组合来表示,那么通常不需要使用自定义算子 API——所有内容(例如 torch.compile
、训练支持)都应该可以正常工作。
示例:将 PIL 的 crop 包装到自定义算子中¶
假设我们正在使用 PIL 的 crop
操作。
import torch
from torchvision.transforms.functional import to_pil_image, pil_to_tensor
import PIL
import IPython
import matplotlib.pyplot as plt
def crop(pic, box):
img = to_pil_image(pic.cpu())
cropped_img = img.crop(box)
return pil_to_tensor(cropped_img).to(pic.device) / 255.
def display(img):
plt.imshow(img.numpy().transpose((1, 2, 0)))
img = torch.ones(3, 64, 64)
img *= torch.linspace(0, 1, steps=64) * torch.linspace(0, 1, steps=64).unsqueeze(-1)
display(img)
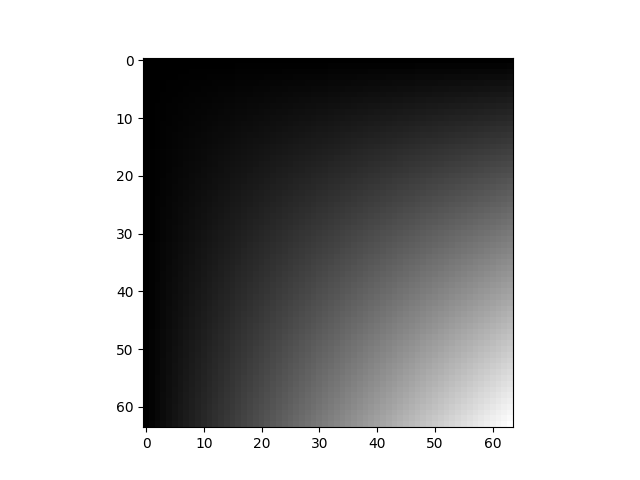
cropped_img = crop(img, (10, 10, 50, 50))
display(cropped_img)
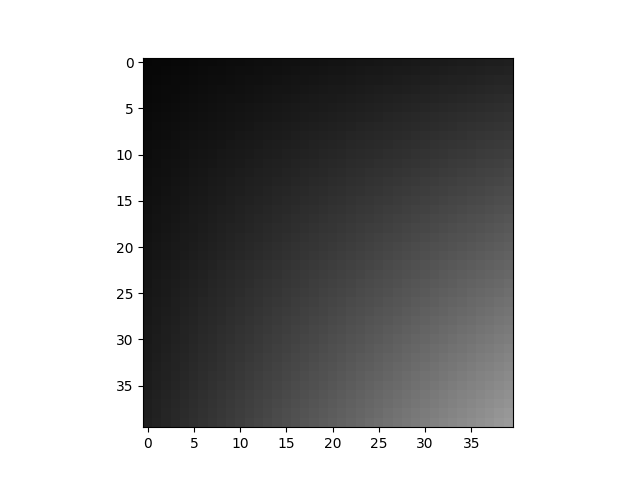
crop
没有被 torch.compile
开箱即用地有效处理:torch.compile
会在它无法处理的函数上引发“图中断”,而图中断不利于性能。以下代码通过引发错误演示了这一点(如果发生图中断,则使用 fullgraph=True
的 torch.compile
会引发错误)。
为了将crop
函数封装成黑盒,以便与torch.compile
一起使用,我们需要做两件事。
将该函数封装成一个PyTorch自定义算子。
为该算子添加一个“
FakeTensor
内核”(也称为“元内核”)。给定一些FakeTensors
输入(不包含存储的虚拟张量),此函数应该返回您选择的虚拟张量,并包含正确的张量元数据(形状/步长/dtype
/设备)。
from typing import Sequence
# Use torch.library.custom_op to define a new custom operator.
# If your operator mutates any input Tensors, their names must be specified
# in the ``mutates_args`` argument.
@torch.library.custom_op("mylib::crop", mutates_args=())
def crop(pic: torch.Tensor, box: Sequence[int]) -> torch.Tensor:
img = to_pil_image(pic.cpu())
cropped_img = img.crop(box)
return (pil_to_tensor(cropped_img) / 255.).to(pic.device, pic.dtype)
# Use register_fake to add a ``FakeTensor`` kernel for the operator
@crop.register_fake
def _(pic, box):
channels = pic.shape[0]
x0, y0, x1, y1 = box
return pic.new_empty(channels, y1 - y0, x1 - x0)
之后,crop
现在可以在不中断计算图的情况下工作。
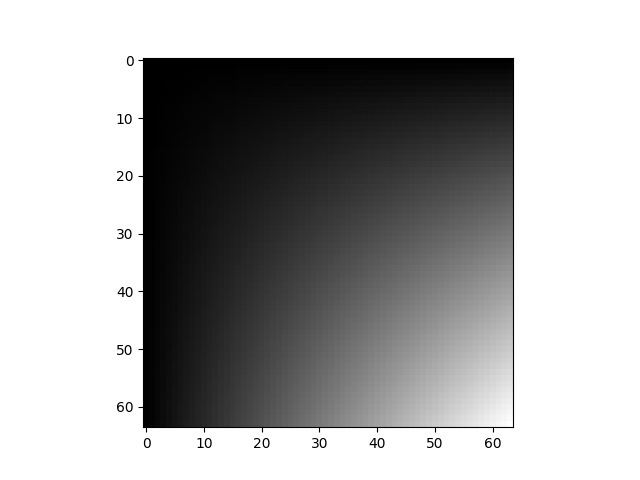
display(cropped_img)
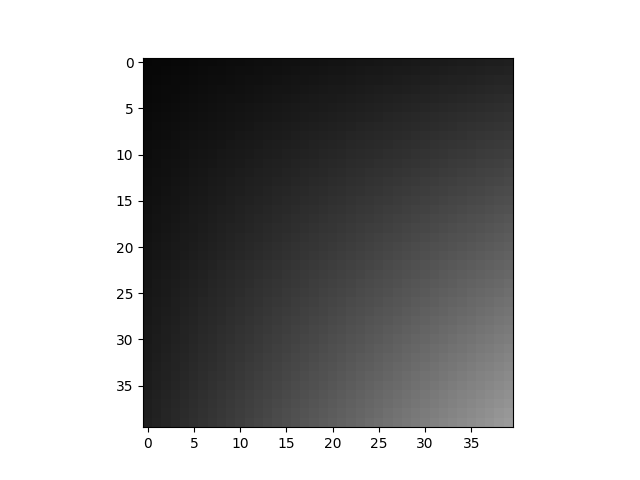
为crop添加训练支持¶
使用torch.library.register_autograd
为算子添加训练支持。优先使用此方法,而不是直接使用torch.autograd.Function
;autograd.Function
与PyTorch算子注册API的某些组合可能导致(并且已经导致)与torch.compile
组合时出现静默错误。
如果您不需要训练支持,则无需使用torch.library.register_autograd
。如果您最终使用没有自动微分注册的custom_op
进行训练,我们将引发错误消息。
crop
的梯度公式本质上是PIL.paste
(我们将推导过程留给读者作为练习)。让我们首先将paste
封装成一个自定义算子。
@torch.library.custom_op("mylib::paste", mutates_args=())
def paste(im1: torch.Tensor, im2: torch.Tensor, coord: Sequence[int]) -> torch.Tensor:
assert im1.device == im2.device
assert im1.dtype == im2.dtype
im1_pil = to_pil_image(im1.cpu())
im2_pil = to_pil_image(im2.cpu())
PIL.Image.Image.paste(im1_pil, im2_pil, coord)
return (pil_to_tensor(im1_pil) / 255.).to(im1.device, im1.dtype)
@paste.register_fake
def _(im1, im2, coord):
assert im1.device == im2.device
assert im1.dtype == im2.dtype
return torch.empty_like(im1)
现在让我们使用register_autograd
为crop
指定梯度公式。
def backward(ctx, grad_output):
grad_input = grad_output.new_zeros(ctx.pic_shape)
grad_input = paste(grad_input, grad_output, ctx.coords)
return grad_input, None
def setup_context(ctx, inputs, output):
pic, box = inputs
ctx.coords = box[:2]
ctx.pic_shape = pic.shape
crop.register_autograd(backward, setup_context=setup_context)
请注意,反向传播必须是PyTorch理解的算子的组合,这就是我们将paste
封装成自定义算子而不是直接使用PIL的paste
的原因。
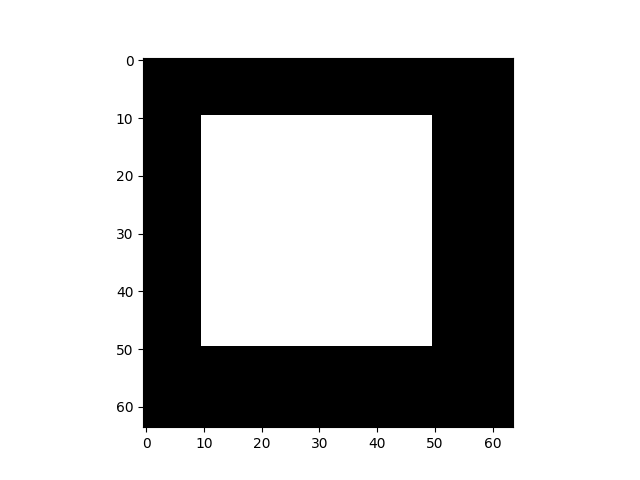
这是正确的梯度,裁剪区域为1(白色),未使用的区域为0(黑色)。
测试Python自定义算子¶
使用torch.library.opcheck
测试自定义算子是否已正确注册。这不会测试梯度在数学上是否正确;请为此编写单独的测试(手动测试或torch.autograd.gradcheck
)。
要使用opcheck
,请向其传递一组示例输入以进行测试。如果您的算子支持训练,则示例应包含需要梯度的张量。如果您的算子支持多个设备,则示例应包含来自每个设备的张量。
examples = [
[torch.randn(3, 64, 64), [0, 0, 10, 10]],
[torch.randn(3, 91, 91, requires_grad=True), [10, 0, 20, 10]],
[torch.randn(3, 60, 60, dtype=torch.double), [3, 4, 32, 20]],
[torch.randn(3, 512, 512, requires_grad=True, dtype=torch.double), [3, 4, 32, 45]],
]
for example in examples:
torch.library.opcheck(crop, example)
可变Python自定义算子¶
您还可以将修改其输入的Python函数封装成自定义算子。修改输入的函数很常见,因为许多底层内核都是这样编写的;例如,计算sin
的内核可能会接收输入和输出张量,并将input.sin()
写入输出张量。
我们将使用numpy.sin
来演示可变Python自定义算子的示例。
import numpy as np
@torch.library.custom_op("mylib::numpy_sin", mutates_args={"output"}, device_types="cpu")
def numpy_sin(input: torch.Tensor, output: torch.Tensor) -> None:
assert input.device == output.device
assert input.device.type == "cpu"
input_np = input.numpy()
output_np = output.numpy()
np.sin(input_np, out=output_np)
由于算子不返回任何内容,因此无需注册FakeTensor
内核(元内核)即可使其与torch.compile
一起使用。
@torch.compile(fullgraph=True)
def f(x):
out = torch.empty(3)
numpy_sin(x, out)
return out
x = torch.randn(3)
y = f(x)
assert torch.allclose(y, x.sin())
这是一个opcheck
运行结果,告诉我们确实已正确注册了算子。opcheck
会在我们忘记将输出添加到mutates_args
时报错,例如。
example_inputs = [
[torch.randn(3), torch.empty(3)],
[torch.randn(0, 3), torch.empty(0, 3)],
[torch.randn(1, 2, 3, 4, dtype=torch.double), torch.empty(1, 2, 3, 4, dtype=torch.double)],
]
for example in example_inputs:
torch.library.opcheck(numpy_sin, example)
结论¶
在本教程中,我们学习了如何使用torch.library.custom_op
在Python中创建自定义算子,该算子可与PyTorch子系统(如torch.compile
和自动微分)一起使用。
本教程提供了对自定义算子的基本介绍。有关更详细的信息,请参阅
脚本总运行时间:(0分钟5.260秒)