注意
转到结尾 下载完整的示例代码。
TorchRL 环境¶
作者: Vincent Moens
环境在强化学习设置中起着至关重要的作用,通常有点类似于监督和无监督设置中的数据集。强化学习社区已经非常熟悉 OpenAI gym API,它提供了一种构建环境、初始化环境和与环境交互的灵活方式。然而,还存在许多其他库,并且与它们的交互方式可能与 gym 的预期大相径庭。
让我们首先描述 TorchRL 如何与 gym 交互,这将作为其他框架的介绍。
Gym 环境¶
要运行本教程的这一部分,您需要安装 gym 库的最新版本以及 atari 套件。您可以通过安装以下软件包来安装它们
为了统一所有框架,torchrl 环境在 __init__
方法中使用名为 _build_env
的私有方法构建,该方法会将参数和关键字参数传递给根库构建器。
对于 gym,这意味着构建环境就像这样简单
import torch
from matplotlib import pyplot as plt
from tensordict import TensorDict
可以通过以下命令访问可用环境的列表
list(GymEnv.available_envs)[:10]
['ALE/Adventure-ram-v5', 'ALE/Adventure-v5', 'ALE/AirRaid-ram-v5', 'ALE/AirRaid-v5', 'ALE/Alien-ram-v5', 'ALE/Alien-v5', 'ALE/Amidar-ram-v5', 'ALE/Amidar-v5', 'ALE/Assault-ram-v5', 'ALE/Assault-v5']
环境规格 (Env Specs)¶
与其他框架一样,TorchRL 环境具有指示观测、动作、完成和奖励空间的属性。由于通常会检索到多个观测,我们期望观测规格为 CompositeSpec
类型。奖励和动作没有此限制
print("Env observation_spec: \n", env.observation_spec)
print("Env action_spec: \n", env.action_spec)
print("Env reward_spec: \n", env.reward_spec)
Env observation_spec:
Composite(
observation: BoundedContinuous(
shape=torch.Size([3]),
space=ContinuousBox(
low=Tensor(shape=torch.Size([3]), device=cpu, dtype=torch.float32, contiguous=True),
high=Tensor(shape=torch.Size([3]), device=cpu, dtype=torch.float32, contiguous=True)),
device=cpu,
dtype=torch.float32,
domain=continuous),
device=None,
shape=torch.Size([]))
Env action_spec:
BoundedContinuous(
shape=torch.Size([1]),
space=ContinuousBox(
low=Tensor(shape=torch.Size([1]), device=cpu, dtype=torch.float32, contiguous=True),
high=Tensor(shape=torch.Size([1]), device=cpu, dtype=torch.float32, contiguous=True)),
device=cpu,
dtype=torch.float32,
domain=continuous)
Env reward_spec:
UnboundedContinuous(
shape=torch.Size([1]),
space=ContinuousBox(
low=Tensor(shape=torch.Size([1]), device=cpu, dtype=torch.float32, contiguous=True),
high=Tensor(shape=torch.Size([1]), device=cpu, dtype=torch.float32, contiguous=True)),
device=cpu,
dtype=torch.float32,
domain=continuous)
这些规格带有一系列有用的工具:可以断言样本是否在定义的空间中。如果样本超出空间,我们还可以使用一些启发式方法将样本投影到空间中,并在该空间中生成随机(可能是均匀分布的)数字
action = torch.ones(1) * 3
print("action is in bounds?\n", bool(env.action_spec.is_in(action)))
print("projected action: \n", env.action_spec.project(action))
action is in bounds?
False
projected action:
tensor([2.])
print("random action: \n", env.action_spec.rand())
random action:
tensor([-1.3541])
在这些规格中,done_spec
值得特别关注。在 TorchRL 中,所有环境至少写入两种类型的轨迹结束信号:"terminated"
(指示马尔可夫决策过程已达到最终状态 - __episode__ 已结束)和 "done"
,指示这是 __trajectory__ 的最后一步(但不一定是任务的结束)。通常,当 "terminal"
为 False
时,"done"
条目为 True
是由 "truncated"
信号引起的。Gym 环境考虑了这三个信号
print(env.done_spec)
Composite(
done: Categorical(
shape=torch.Size([1]),
space=CategoricalBox(n=2),
device=cpu,
dtype=torch.bool,
domain=discrete),
terminated: Categorical(
shape=torch.Size([1]),
space=CategoricalBox(n=2),
device=cpu,
dtype=torch.bool,
domain=discrete),
truncated: Categorical(
shape=torch.Size([1]),
space=CategoricalBox(n=2),
device=cpu,
dtype=torch.bool,
domain=discrete),
device=None,
shape=torch.Size([]))
环境还包含一个类型为 CompositeSpec
的 env.state_spec
属性,其中包含环境的输入的所有规格,但不是动作。对于有状态环境(例如 gym),这在大多数情况下将是空的。对于无状态环境(例如 Brax),这还应包括先前状态的表示,或环境的任何其他输入(包括重置时的输入)。
播种、重置和步进 (Seeding, resetting and steps)¶
环境上的基本操作是 (1) set_seed
,(2) reset
和 (3) step
。
让我们看看这些方法如何在 TorchRL 中工作
torch.manual_seed(0) # make sure that all torch code is also reproductible
env.set_seed(0)
reset_data = env.reset()
print("reset data", reset_data)
reset data TensorDict(
fields={
done: Tensor(shape=torch.Size([1]), device=cpu, dtype=torch.bool, is_shared=False),
observation: Tensor(shape=torch.Size([3]), device=cpu, dtype=torch.float32, is_shared=False),
terminated: Tensor(shape=torch.Size([1]), device=cpu, dtype=torch.bool, is_shared=False),
truncated: Tensor(shape=torch.Size([1]), device=cpu, dtype=torch.bool, is_shared=False)},
batch_size=torch.Size([]),
device=None,
is_shared=False)
我们现在可以在环境中执行一步。由于我们没有策略,我们可以只生成一个随机动作
policy = TensorDictModule(env.action_spec.rand, in_keys=[], out_keys=["action"])
policy(reset_data)
tensordict_out = env.step(reset_data)
默认情况下,step
返回的 tensordict 与输入相同……
assert tensordict_out is reset_data
……但带有新键
tensordict_out
TensorDict(
fields={
action: Tensor(shape=torch.Size([1]), device=cpu, dtype=torch.float32, is_shared=False),
done: Tensor(shape=torch.Size([1]), device=cpu, dtype=torch.bool, is_shared=False),
next: TensorDict(
fields={
done: Tensor(shape=torch.Size([1]), device=cpu, dtype=torch.bool, is_shared=False),
observation: Tensor(shape=torch.Size([3]), device=cpu, dtype=torch.float32, is_shared=False),
reward: Tensor(shape=torch.Size([1]), device=cpu, dtype=torch.float32, is_shared=False),
terminated: Tensor(shape=torch.Size([1]), device=cpu, dtype=torch.bool, is_shared=False),
truncated: Tensor(shape=torch.Size([1]), device=cpu, dtype=torch.bool, is_shared=False)},
batch_size=torch.Size([]),
device=None,
is_shared=False),
observation: Tensor(shape=torch.Size([3]), device=cpu, dtype=torch.float32, is_shared=False),
terminated: Tensor(shape=torch.Size([1]), device=cpu, dtype=torch.bool, is_shared=False),
truncated: Tensor(shape=torch.Size([1]), device=cpu, dtype=torch.bool, is_shared=False)},
batch_size=torch.Size([]),
device=None,
is_shared=False)
我们刚刚所做的(使用 action_spec.rand()
的随机步进)也可以通过简单的快捷方式完成。
env.rand_step()
TensorDict(
fields={
action: Tensor(shape=torch.Size([1]), device=cpu, dtype=torch.float32, is_shared=False),
next: TensorDict(
fields={
done: Tensor(shape=torch.Size([1]), device=cpu, dtype=torch.bool, is_shared=False),
observation: Tensor(shape=torch.Size([3]), device=cpu, dtype=torch.float32, is_shared=False),
reward: Tensor(shape=torch.Size([1]), device=cpu, dtype=torch.float32, is_shared=False),
terminated: Tensor(shape=torch.Size([1]), device=cpu, dtype=torch.bool, is_shared=False),
truncated: Tensor(shape=torch.Size([1]), device=cpu, dtype=torch.bool, is_shared=False)},
batch_size=torch.Size([]),
device=None,
is_shared=False)},
batch_size=torch.Size([]),
device=None,
is_shared=False)
新键 ("next", "observation")
(以及 "next"
tensordict 下的所有键)在 TorchRL 中具有特殊作用:它们指示它们在具有相同名称但没有前缀的键之后出现。
我们提供了一个函数 step_mdp
,它在 tensordict 中执行一步:它返回一个新的 tensordict,更新后使得 *t < -t’*
from torchrl.envs.utils import step_mdp
tensordict_out.set("some other key", torch.randn(1))
tensordict_tprime = step_mdp(tensordict_out)
print(tensordict_tprime)
print(
(
tensordict_tprime.get("observation")
== tensordict_out.get(("next", "observation"))
).all()
)
TensorDict(
fields={
done: Tensor(shape=torch.Size([1]), device=cpu, dtype=torch.bool, is_shared=False),
observation: Tensor(shape=torch.Size([3]), device=cpu, dtype=torch.float32, is_shared=False),
some other key: Tensor(shape=torch.Size([1]), device=cpu, dtype=torch.float32, is_shared=False),
terminated: Tensor(shape=torch.Size([1]), device=cpu, dtype=torch.bool, is_shared=False),
truncated: Tensor(shape=torch.Size([1]), device=cpu, dtype=torch.bool, is_shared=False)},
batch_size=torch.Size([]),
device=None,
is_shared=False)
tensor(True)
我们可以观察到 step_mdp
已删除所有时间相关的键值对,但不包括 "some other key"
。此外,新的观测与之前的观测相匹配。
最后,请注意 env.reset
方法也接受要更新的 tensordict
tensordict = TensorDict({}, [])
assert env.reset(tensordict) is tensordict
tensordict
TensorDict(
fields={
done: Tensor(shape=torch.Size([1]), device=cpu, dtype=torch.bool, is_shared=False),
observation: Tensor(shape=torch.Size([3]), device=cpu, dtype=torch.float32, is_shared=False),
terminated: Tensor(shape=torch.Size([1]), device=cpu, dtype=torch.bool, is_shared=False),
truncated: Tensor(shape=torch.Size([1]), device=cpu, dtype=torch.bool, is_shared=False)},
batch_size=torch.Size([]),
device=None,
is_shared=False)
Rollouts¶
TorchRL 提供的通用环境类允许您轻松地为给定步数运行 rollouts
tensordict_rollout = env.rollout(max_steps=20, policy=policy)
print(tensordict_rollout)
TensorDict(
fields={
action: Tensor(shape=torch.Size([20, 1]), device=cpu, dtype=torch.float32, is_shared=False),
done: Tensor(shape=torch.Size([20, 1]), device=cpu, dtype=torch.bool, is_shared=False),
next: TensorDict(
fields={
done: Tensor(shape=torch.Size([20, 1]), device=cpu, dtype=torch.bool, is_shared=False),
observation: Tensor(shape=torch.Size([20, 3]), device=cpu, dtype=torch.float32, is_shared=False),
reward: Tensor(shape=torch.Size([20, 1]), device=cpu, dtype=torch.float32, is_shared=False),
terminated: Tensor(shape=torch.Size([20, 1]), device=cpu, dtype=torch.bool, is_shared=False),
truncated: Tensor(shape=torch.Size([20, 1]), device=cpu, dtype=torch.bool, is_shared=False)},
batch_size=torch.Size([20]),
device=None,
is_shared=False),
observation: Tensor(shape=torch.Size([20, 3]), device=cpu, dtype=torch.float32, is_shared=False),
terminated: Tensor(shape=torch.Size([20, 1]), device=cpu, dtype=torch.bool, is_shared=False),
truncated: Tensor(shape=torch.Size([20, 1]), device=cpu, dtype=torch.bool, is_shared=False)},
batch_size=torch.Size([20]),
device=None,
is_shared=False)
生成的 tensordict 的 batch_size
为 [20]
,即轨迹的长度。我们可以检查观测是否与其下一个值匹配
(
tensordict_rollout.get("observation")[1:]
== tensordict_rollout.get(("next", "observation"))[:-1]
).all()
tensor(True)
frame_skip
¶
在某些情况下,使用 frame_skip
参数对多个连续帧使用相同的动作很有用。
生成的 tensordict 将仅包含序列中观察到的最后一帧,但奖励将会在帧数上求和。
如果环境在此过程中达到完成状态,它将停止并返回截断链的结果。
env = GymEnv("Pendulum-v1", frame_skip=4)
env.reset()
TensorDict(
fields={
done: Tensor(shape=torch.Size([1]), device=cpu, dtype=torch.bool, is_shared=False),
observation: Tensor(shape=torch.Size([3]), device=cpu, dtype=torch.float32, is_shared=False),
terminated: Tensor(shape=torch.Size([1]), device=cpu, dtype=torch.bool, is_shared=False),
truncated: Tensor(shape=torch.Size([1]), device=cpu, dtype=torch.bool, is_shared=False)},
batch_size=torch.Size([]),
device=None,
is_shared=False)
渲染 (Rendering)¶
渲染在许多强化学习设置中起着重要作用,这就是为什么 torchrl 中的通用环境类提供 from_pixels
关键字参数,允许用户快速请求基于图像的环境
env = GymEnv("Pendulum-v1", from_pixels=True)
tensordict = env.reset()
env.close()
plt.imshow(tensordict.get("pixels").numpy())
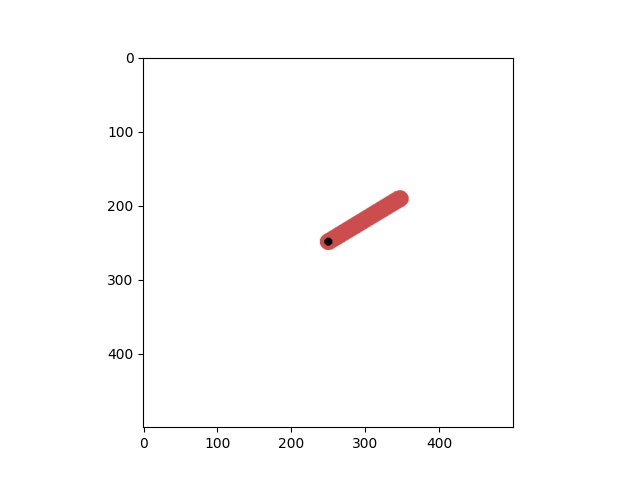
<matplotlib.image.AxesImage object at 0x7fb1c4b85e10>
让我们看看 tensordict 包含什么
tensordict
TensorDict(
fields={
done: Tensor(shape=torch.Size([1]), device=cpu, dtype=torch.bool, is_shared=False),
pixels: Tensor(shape=torch.Size([500, 500, 3]), device=cpu, dtype=torch.uint8, is_shared=False),
terminated: Tensor(shape=torch.Size([1]), device=cpu, dtype=torch.bool, is_shared=False),
truncated: Tensor(shape=torch.Size([1]), device=cpu, dtype=torch.bool, is_shared=False)},
batch_size=torch.Size([]),
device=None,
is_shared=False)
我们仍然有一个 "state"
,它描述了 "observation"
在之前的情况下用于描述的内容(命名差异来自 gym 现在返回一个字典,而 TorchRL 从字典中获取名称,如果字典存在,否则它将步进输出命名为 "observation"
:简而言之,这是由于 gym 环境步进方法返回的对象类型不一致造成的)。
也可以通过仅请求像素来丢弃此补充输出
env = GymEnv("Pendulum-v1", from_pixels=True, pixels_only=True)
env.reset()
env.close()
某些环境仅以基于图像的格式提供
env = GymEnv("ALE/Pong-v5")
print("from pixels: ", env.from_pixels)
print("tensordict: ", env.reset())
env.close()
from pixels: True
tensordict: TensorDict(
fields={
done: Tensor(shape=torch.Size([1]), device=cpu, dtype=torch.bool, is_shared=False),
pixels: Tensor(shape=torch.Size([210, 160, 3]), device=cpu, dtype=torch.uint8, is_shared=False),
terminated: Tensor(shape=torch.Size([1]), device=cpu, dtype=torch.bool, is_shared=False),
truncated: Tensor(shape=torch.Size([1]), device=cpu, dtype=torch.bool, is_shared=False)},
batch_size=torch.Size([]),
device=None,
is_shared=False)
DeepMind Control 环境¶
- 要运行本教程的这一部分,请确保您已安装 dm_control
$ pip install dm_control
我们还为 DM Control 套件提供了一个包装器。同样,构建环境也很容易:首先让我们看看可以访问哪些环境。available_envs
现在返回一个环境和可能任务的字典
from matplotlib import pyplot as plt
from torchrl.envs.libs.dm_control import DMControlEnv
DMControlEnv.available_envs
[('acrobot', ['swingup', 'swingup_sparse']), ('ball_in_cup', ['catch']), ('cartpole', ['balance', 'balance_sparse', 'swingup', 'swingup_sparse', 'three_poles', 'two_poles']), ('cheetah', ['run']), ('finger', ['spin', 'turn_easy', 'turn_hard']), ('fish', ['upright', 'swim']), ('hopper', ['stand', 'hop']), ('humanoid', ['stand', 'walk', 'run', 'run_pure_state']), ('manipulator', ['bring_ball', 'bring_peg', 'insert_ball', 'insert_peg']), ('pendulum', ['swingup']), ('point_mass', ['easy', 'hard']), ('reacher', ['easy', 'hard']), ('swimmer', ['swimmer6', 'swimmer15']), ('walker', ['stand', 'walk', 'run']), ('dog', ['fetch', 'run', 'stand', 'trot', 'walk']), ('humanoid_CMU', ['run', 'stand', 'walk']), ('lqr', ['lqr_2_1', 'lqr_6_2']), ('quadruped', ['escape', 'fetch', 'run', 'walk']), ('stacker', ['stack_2', 'stack_4'])]
env = DMControlEnv("acrobot", "swingup")
tensordict = env.reset()
print("result of reset: ", tensordict)
env.close()
result of reset: TensorDict(
fields={
done: Tensor(shape=torch.Size([1]), device=cpu, dtype=torch.bool, is_shared=False),
orientations: Tensor(shape=torch.Size([4]), device=cpu, dtype=torch.float64, is_shared=False),
terminated: Tensor(shape=torch.Size([1]), device=cpu, dtype=torch.bool, is_shared=False),
truncated: Tensor(shape=torch.Size([1]), device=cpu, dtype=torch.bool, is_shared=False),
velocity: Tensor(shape=torch.Size([2]), device=cpu, dtype=torch.float64, is_shared=False)},
batch_size=torch.Size([]),
device=None,
is_shared=False)
当然,我们也可以使用基于像素的环境
env = DMControlEnv("acrobot", "swingup", from_pixels=True, pixels_only=True)
tensordict = env.reset()
print("result of reset: ", tensordict)
plt.imshow(tensordict.get("pixels").numpy())
env.close()
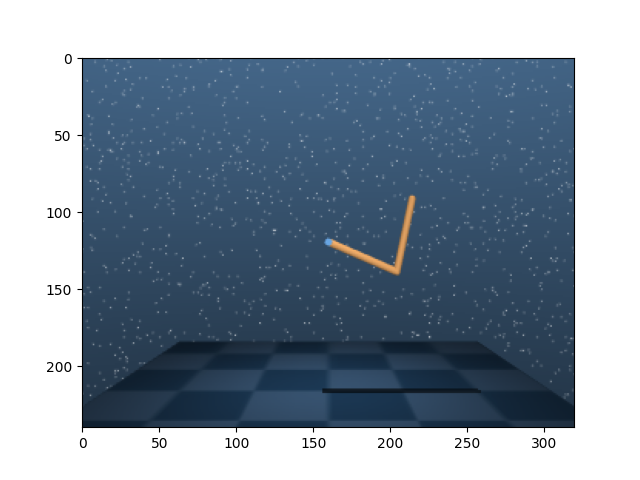
result of reset: TensorDict(
fields={
done: Tensor(shape=torch.Size([1]), device=cpu, dtype=torch.bool, is_shared=False),
pixels: Tensor(shape=torch.Size([240, 320, 3]), device=cpu, dtype=torch.uint8, is_shared=False),
terminated: Tensor(shape=torch.Size([1]), device=cpu, dtype=torch.bool, is_shared=False),
truncated: Tensor(shape=torch.Size([1]), device=cpu, dtype=torch.bool, is_shared=False)},
batch_size=torch.Size([]),
device=None,
is_shared=False)
转换环境 (Transforming envs)¶
在许多情况下,通常在策略读取或存储到缓冲区之前预处理环境的输出。
- 在许多情况下,强化学习社区采用了以下类型的包装方案
$ env_transformed = wrapper1(wrapper2(env))
来转换环境。这有很多优点:它使访问环境规格变得显而易见(外部包装器是外部世界的真理来源),并且易于与向量化环境交互。然而,它也使得访问内部环境变得困难:假设有人想从链中删除包装器(例如 wrapper2
),此操作需要我们收集
$ env0 = env.env.env
$ env_transformed_bis = wrapper1(env0)
TorchRL 采取使用转换序列的立场,就像在其他 pytorch 域库(例如 torchvision
)中所做的那样。这种方法也类似于在 torch.distribution
中转换分布的方式,其中 TransformedDistribution
对象围绕 base_dist
分布和(转换)transforms
序列构建。
from torchrl.envs.transforms import ToTensorImage, TransformedEnv
# ToTensorImage transforms a numpy-like image into a tensor one,
env = DMControlEnv("acrobot", "swingup", from_pixels=True, pixels_only=True)
print("reset before transform: ", env.reset())
env = TransformedEnv(env, ToTensorImage())
print("reset after transform: ", env.reset())
env.close()
reset before transform: TensorDict(
fields={
done: Tensor(shape=torch.Size([1]), device=cpu, dtype=torch.bool, is_shared=False),
pixels: Tensor(shape=torch.Size([240, 320, 3]), device=cpu, dtype=torch.uint8, is_shared=False),
terminated: Tensor(shape=torch.Size([1]), device=cpu, dtype=torch.bool, is_shared=False),
truncated: Tensor(shape=torch.Size([1]), device=cpu, dtype=torch.bool, is_shared=False)},
batch_size=torch.Size([]),
device=None,
is_shared=False)
reset after transform: TensorDict(
fields={
done: Tensor(shape=torch.Size([1]), device=cpu, dtype=torch.bool, is_shared=False),
pixels: Tensor(shape=torch.Size([3, 240, 320]), device=cpu, dtype=torch.float32, is_shared=False),
terminated: Tensor(shape=torch.Size([1]), device=cpu, dtype=torch.bool, is_shared=False),
truncated: Tensor(shape=torch.Size([1]), device=cpu, dtype=torch.bool, is_shared=False)},
batch_size=torch.Size([]),
device=None,
is_shared=False)
要组合转换,只需使用 Compose
类
from torchrl.envs.transforms import Compose, Resize
env = DMControlEnv("acrobot", "swingup", from_pixels=True, pixels_only=True)
env = TransformedEnv(env, Compose(ToTensorImage(), Resize(32, 32)))
env.reset()
TensorDict(
fields={
done: Tensor(shape=torch.Size([1]), device=cpu, dtype=torch.bool, is_shared=False),
pixels: Tensor(shape=torch.Size([3, 32, 32]), device=cpu, dtype=torch.float32, is_shared=False),
terminated: Tensor(shape=torch.Size([1]), device=cpu, dtype=torch.bool, is_shared=False),
truncated: Tensor(shape=torch.Size([1]), device=cpu, dtype=torch.bool, is_shared=False)},
batch_size=torch.Size([]),
device=None,
is_shared=False)
也可以一次添加一个转换
TensorDict(
fields={
done: Tensor(shape=torch.Size([1]), device=cpu, dtype=torch.bool, is_shared=False),
pixels: Tensor(shape=torch.Size([1, 32, 32]), device=cpu, dtype=torch.float32, is_shared=False),
terminated: Tensor(shape=torch.Size([1]), device=cpu, dtype=torch.bool, is_shared=False),
truncated: Tensor(shape=torch.Size([1]), device=cpu, dtype=torch.bool, is_shared=False)},
batch_size=torch.Size([]),
device=None,
is_shared=False)
正如预期的那样,元数据也会更新
print("original obs spec: ", env.base_env.observation_spec)
print("current obs spec: ", env.observation_spec)
original obs spec: Composite(
pixels: UnboundedDiscrete(
shape=torch.Size([240, 320, 3]),
space=ContinuousBox(
low=Tensor(shape=torch.Size([240, 320, 3]), device=cpu, dtype=torch.uint8, contiguous=True),
high=Tensor(shape=torch.Size([240, 320, 3]), device=cpu, dtype=torch.uint8, contiguous=True)),
device=cpu,
dtype=torch.uint8,
domain=discrete),
device=None,
shape=torch.Size([]))
current obs spec: Composite(
pixels: UnboundedContinuous(
shape=torch.Size([1, 32, 32]),
space=ContinuousBox(
low=Tensor(shape=torch.Size([1, 32, 32]), device=cpu, dtype=torch.float32, contiguous=True),
high=Tensor(shape=torch.Size([1, 32, 32]), device=cpu, dtype=torch.float32, contiguous=True)),
device=cpu,
dtype=torch.float32,
domain=continuous),
device=None,
shape=torch.Size([]))
如果需要,我们也可以连接张量
from torchrl.envs.transforms import CatTensors
env = DMControlEnv("acrobot", "swingup")
print("keys before concat: ", env.reset())
env = TransformedEnv(
env,
CatTensors(in_keys=["orientations", "velocity"], out_key="observation"),
)
print("keys after concat: ", env.reset())
keys before concat: TensorDict(
fields={
done: Tensor(shape=torch.Size([1]), device=cpu, dtype=torch.bool, is_shared=False),
orientations: Tensor(shape=torch.Size([4]), device=cpu, dtype=torch.float64, is_shared=False),
terminated: Tensor(shape=torch.Size([1]), device=cpu, dtype=torch.bool, is_shared=False),
truncated: Tensor(shape=torch.Size([1]), device=cpu, dtype=torch.bool, is_shared=False),
velocity: Tensor(shape=torch.Size([2]), device=cpu, dtype=torch.float64, is_shared=False)},
batch_size=torch.Size([]),
device=None,
is_shared=False)
keys after concat: TensorDict(
fields={
done: Tensor(shape=torch.Size([1]), device=cpu, dtype=torch.bool, is_shared=False),
observation: Tensor(shape=torch.Size([6]), device=cpu, dtype=torch.float64, is_shared=False),
terminated: Tensor(shape=torch.Size([1]), device=cpu, dtype=torch.bool, is_shared=False),
truncated: Tensor(shape=torch.Size([1]), device=cpu, dtype=torch.bool, is_shared=False)},
batch_size=torch.Size([]),
device=None,
is_shared=False)
此功能使得轻松修改应用于环境输入和输出的转换集。实际上,转换在步进执行之前和之后都会运行:对于预步进传递,in_keys_inv
键列表将传递给 _inv_apply_transform
方法。这种转换的一个示例是将浮点动作(来自神经网络的输出)转换为 double dtype(包装环境需要)。在执行步进后,_apply_transform
方法将在 in_keys
键列表指示的键上执行。
环境转换的另一个有趣的功能是,它们允许用户检索包装情况下的 env.env
等效项,或者换句话说,父环境。可以通过调用 transform.parent
来检索父环境:返回的环境将包含一个 TransformedEnvironment
,其中包含所有转换,直到(但不包括)当前转换。这可以用于例如 NoopResetEnv
情况,当重置时,它执行以下步骤:在环境中随机执行一定数量的步进之前,重置父环境。
env = DMControlEnv("acrobot", "swingup")
env = TransformedEnv(env)
env.append_transform(
CatTensors(in_keys=["orientations", "velocity"], out_key="observation")
)
env.append_transform(GrayScale())
print("env: \n", env)
print("GrayScale transform parent env: \n", env.transform[1].parent)
print("CatTensors transform parent env: \n", env.transform[0].parent)
env:
TransformedEnv(
env=DMControlEnv(env=acrobot, task=swingup, batch_size=torch.Size([])),
transform=Compose(
CatTensors(in_keys=['orientations', 'velocity'], out_key=observation),
GrayScale(keys=['pixels'])))
GrayScale transform parent env:
TransformedEnv(
env=DMControlEnv(env=acrobot, task=swingup, batch_size=torch.Size([])),
transform=Compose(
CatTensors(in_keys=['orientations', 'velocity'], out_key=observation)))
CatTensors transform parent env:
TransformedEnv(
env=DMControlEnv(env=acrobot, task=swingup, batch_size=torch.Size([])),
transform=Compose(
))
环境设备 (Environment device)¶
转换可以在设备上工作,当操作适度或高度计算密集时,可以带来显着的加速。这些包括 ToTensorImage
、Resize
、GrayScale
等。
有人可能会合理地问,这在包装环境方面意味着什么。对于常规环境来说,几乎没有:操作仍将在它们应该发生的设备上发生。torchrl 中的环境设备属性指示传入数据应该在哪个设备上,以及输出数据将在哪个设备上。从该设备到该设备的转换是 torchrl 环境类的责任。在 GPU 上存储数据的主要优势是 (1) 如上所述的转换加速和 (2) 在多处理设置中工作进程之间共享数据。
from torchrl.envs.transforms import CatTensors, GrayScale, TransformedEnv
env = DMControlEnv("acrobot", "swingup")
env = TransformedEnv(env)
env.append_transform(
CatTensors(in_keys=["orientations", "velocity"], out_key="observation")
)
if torch.has_cuda and torch.cuda.device_count():
env.to("cuda:0")
env.reset()
并行运行环境 (Running environments in parallel)¶
TorchRL 提供了并行运行环境的实用程序。预计各种环境读取和返回形状和 dtype 相似的张量(但可以设计掩码函数,以便在这些张量形状不同的情况下使其成为可能)。创建此类环境非常容易。让我们看看最简单的情况
from torchrl.envs import ParallelEnv
def env_make():
return GymEnv("Pendulum-v1")
parallel_env = ParallelEnv(3, env_make) # -> creates 3 envs in parallel
parallel_env = ParallelEnv(
3, [env_make, env_make, env_make]
) # similar to the previous command
SerialEnv
类类似于 ParallelEnv
,不同之处在于环境是按顺序运行的。这主要用于调试目的。
ParallelEnv
实例以惰性模式创建:环境仅在调用时才开始运行。这允许我们在进程之间移动 ParallelEnv
对象,而无需过多担心运行进程。ParallelEnv
可以通过调用 start
、reset
或仅通过调用 step
来启动(如果不需要先调用 reset
)。
parallel_env.reset()
TensorDict(
fields={
done: Tensor(shape=torch.Size([3, 1]), device=cpu, dtype=torch.bool, is_shared=False),
observation: Tensor(shape=torch.Size([3, 3]), device=cpu, dtype=torch.float32, is_shared=False),
terminated: Tensor(shape=torch.Size([3, 1]), device=cpu, dtype=torch.bool, is_shared=False),
truncated: Tensor(shape=torch.Size([3, 1]), device=cpu, dtype=torch.bool, is_shared=False)},
batch_size=torch.Size([3]),
device=None,
is_shared=False)
可以检查并行环境是否具有正确的批大小。按照惯例,batch_size
的第一部分指示批次,第二部分指示时间帧。让我们使用 rollout
方法检查一下
parallel_env.rollout(max_steps=20)
TensorDict(
fields={
action: Tensor(shape=torch.Size([3, 20, 1]), device=cpu, dtype=torch.float32, is_shared=False),
done: Tensor(shape=torch.Size([3, 20, 1]), device=cpu, dtype=torch.bool, is_shared=False),
next: TensorDict(
fields={
done: Tensor(shape=torch.Size([3, 20, 1]), device=cpu, dtype=torch.bool, is_shared=False),
observation: Tensor(shape=torch.Size([3, 20, 3]), device=cpu, dtype=torch.float32, is_shared=False),
reward: Tensor(shape=torch.Size([3, 20, 1]), device=cpu, dtype=torch.float32, is_shared=False),
terminated: Tensor(shape=torch.Size([3, 20, 1]), device=cpu, dtype=torch.bool, is_shared=False),
truncated: Tensor(shape=torch.Size([3, 20, 1]), device=cpu, dtype=torch.bool, is_shared=False)},
batch_size=torch.Size([3, 20]),
device=None,
is_shared=False),
observation: Tensor(shape=torch.Size([3, 20, 3]), device=cpu, dtype=torch.float32, is_shared=False),
terminated: Tensor(shape=torch.Size([3, 20, 1]), device=cpu, dtype=torch.bool, is_shared=False),
truncated: Tensor(shape=torch.Size([3, 20, 1]), device=cpu, dtype=torch.bool, is_shared=False)},
batch_size=torch.Size([3, 20]),
device=None,
is_shared=False)
关闭并行环境 (Closing parallel environments)¶
重要提示:在关闭程序之前,关闭并行环境非常重要。通常,即使使用常规环境,最好也通过调用 close
来关闭函数。在某些情况下,如果不这样做,TorchRL 将抛出错误(并且通常会在程序结束时发生,当环境超出范围时!)
parallel_env.close()
播种 (Seeding)¶
在播种并行环境时,我们面临的困难是我们不想为所有环境提供相同的种子。TorchRL 使用的启发式方法是,我们以马尔可夫方式生成给定输入种子的确定性种子链,以便可以从其任何元素重建该链。所有 set_seed
方法都将返回要使用的下一个种子,以便可以轻松地保持链的进行,给定最后一个种子。当多个收集器都包含 ParallelEnv
实例,并且我们希望每个子子环境都具有不同的种子时,这非常有用。
out_seed = parallel_env.set_seed(10)
print(out_seed)
del parallel_env
3288080526
访问环境属性 (Accessing environment attributes)¶
有时会发生包装环境具有感兴趣的属性。首先,请注意 TorchRL 环境包装器包含访问此属性的工具。这是一个例子
from time import sleep
from uuid import uuid1
def env_make():
env = GymEnv("Pendulum-v1")
env._env.foo = f"bar_{uuid1()}"
env._env.get_something = lambda r: r + 1
return env
env = env_make()
# Goes through env._env
env.foo
'bar_c6313b92-90c7-11ef-a49b-0242ac110002'
parallel_env = ParallelEnv(3, env_make) # -> creates 3 envs in parallel
# env has not been started --> error:
try:
parallel_env.foo
except RuntimeError:
print("Aargh what did I do!")
sleep(2) # make sure we don't get ahead of ourselves
Aargh what did I do!
if parallel_env.is_closed:
parallel_env.start()
foo_list = parallel_env.foo
foo_list # needs to be instantiated, for instance using list
<torchrl.envs.batched_envs._dispatch_caller_parallel object at 0x7fb1c4b87a30>
list(foo_list)
['bar_caa4671c-90c7-11ef-a4ef-0242ac110002', 'bar_ca9e607e-90c7-11ef-bf1d-0242ac110002', 'bar_caa59c2c-90c7-11ef-95b4-0242ac110002']
同样,也可以访问方法
something = parallel_env.get_something(0)
print(something)
[1, 1, 1]
parallel_env.close()
del parallel_env
并行环境的 kwargs (kwargs for parallel environments)¶
可能希望为各种环境提供 kwargs。这可以在构建时或之后实现
from torchrl.envs import ParallelEnv
def env_make(env_name):
env = TransformedEnv(
GymEnv(env_name, from_pixels=True, pixels_only=True),
Compose(ToTensorImage(), Resize(64, 64)),
)
return env
parallel_env = ParallelEnv(
2,
[env_make, env_make],
create_env_kwargs=[{"env_name": "ALE/AirRaid-v5"}, {"env_name": "ALE/Pong-v5"}],
)
tensordict = parallel_env.reset()
plt.figure()
plt.subplot(121)
plt.imshow(tensordict[0].get("pixels").permute(1, 2, 0).numpy())
plt.subplot(122)
plt.imshow(tensordict[1].get("pixels").permute(1, 2, 0).numpy())
parallel_env.close()
del parallel_env
from matplotlib import pyplot as plt
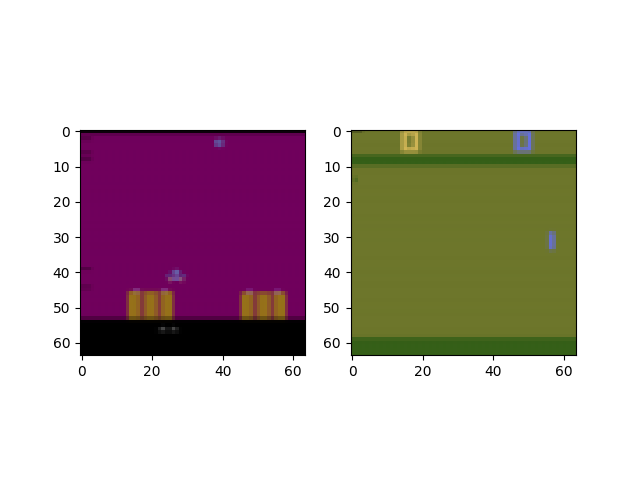
转换并行环境 (Transforming parallel environments)¶
有两种等效的方法来转换并行环境:在每个进程中分别转换,或在主进程中转换。甚至可以两者都做。因此,可以仔细考虑转换设计,以利用设备功能(例如,cuda 设备上的转换)并在可能的情况下向量化主进程上的操作。
from torchrl.envs import (
Compose,
GrayScale,
ParallelEnv,
Resize,
ToTensorImage,
TransformedEnv,
)
def env_make(env_name):
env = TransformedEnv(
GymEnv(env_name, from_pixels=True, pixels_only=True),
Compose(ToTensorImage(), Resize(64, 64)),
) # transforms on remote processes
return env
parallel_env = ParallelEnv(
2,
[env_make, env_make],
create_env_kwargs=[{"env_name": "ALE/AirRaid-v5"}, {"env_name": "ALE/Pong-v5"}],
)
parallel_env = TransformedEnv(parallel_env, GrayScale()) # transforms on main process
tensordict = parallel_env.reset()
print("grayscale tensordict: ", tensordict)
plt.figure()
plt.subplot(121)
plt.imshow(tensordict[0].get("pixels").permute(1, 2, 0).numpy())
plt.subplot(122)
plt.imshow(tensordict[1].get("pixels").permute(1, 2, 0).numpy())
parallel_env.close()
del parallel_env
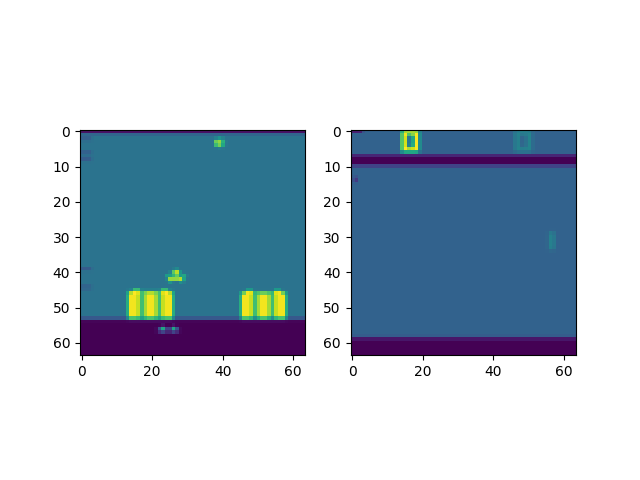
grayscale tensordict: TensorDict(
fields={
done: Tensor(shape=torch.Size([2, 1]), device=cpu, dtype=torch.bool, is_shared=False),
pixels: Tensor(shape=torch.Size([2, 1, 64, 64]), device=cpu, dtype=torch.float32, is_shared=False),
terminated: Tensor(shape=torch.Size([2, 1]), device=cpu, dtype=torch.bool, is_shared=False),
truncated: Tensor(shape=torch.Size([2, 1]), device=cpu, dtype=torch.bool, is_shared=False)},
batch_size=torch.Size([2]),
device=None,
is_shared=False)
VecNorm¶
在强化学习中,我们通常面临在将数据输入模型之前对其进行归一化的问题。有时,我们可以从环境中收集的数据中获得归一化统计信息的良好近似值,例如,使用随机策略(或演示)。然而,最好“动态”地归一化数据,逐步更新归一化常数以适应迄今为止观察到的情况。当我们预期归一化统计信息会随着任务性能的变化而变化,或者当环境由于外部因素而演变时,这尤其有用。
注意:在离线策略学习中应谨慎使用此功能,因为旧数据将因其使用先前有效的归一化统计信息进行归一化而被“弃用”。在在线策略设置中,此功能也会使学习变得不稳定,并可能产生意外的影响。因此,建议用户谨慎依赖此功能,并将其与给定固定版本归一化常数的数据归一化进行比较。
在常规设置中,使用 VecNorm 非常容易
from torchrl.envs.libs.gym import GymEnv
from torchrl.envs.transforms import TransformedEnv, VecNorm
env = TransformedEnv(GymEnv("Pendulum-v1"), VecNorm())
tensordict = env.rollout(max_steps=100)
print("mean: :", tensordict.get("observation").mean(0)) # Approx 0
print("std: :", tensordict.get("observation").std(0)) # Approx 1
mean: : tensor([-0.1122, 0.2134, -0.1901])
std: : tensor([1.1596, 1.1628, 1.0870])
在并行环境中,事情稍微复杂一些,因为我们需要在进程之间共享运行统计信息。我们创建了一个类 EnvCreator
,它负责查看环境创建方法,检索要在环境类中的进程之间共享的 tensordict,并在创建后将每个进程指向正确的公共共享 tensordict
from torchrl.envs import EnvCreator, ParallelEnv
from torchrl.envs.libs.gym import GymEnv
from torchrl.envs.transforms import TransformedEnv, VecNorm
make_env = EnvCreator(lambda: TransformedEnv(GymEnv("CartPole-v1"), VecNorm(decay=1.0)))
env = ParallelEnv(3, make_env)
make_env.state_dict()["_extra_state"]["td"]["observation_count"].fill_(0.0)
make_env.state_dict()["_extra_state"]["td"]["observation_ssq"].fill_(0.0)
make_env.state_dict()["_extra_state"]["td"]["observation_sum"].fill_(0.0)
tensordict = env.rollout(max_steps=5)
print("tensordict: ", tensordict)
print("mean: :", tensordict.get("observation").view(-1, 3).mean(0)) # Approx 0
print("std: :", tensordict.get("observation").view(-1, 3).std(0)) # Approx 1
Traceback (most recent call last):
File "/pytorch/rl/docs/source/reference/generated/tutorials/torchrl_envs.py", line 697, in <module>
make_env.state_dict()["_extra_state"]["td"]["observation_count"].fill_(0.0)
KeyError: 'td'
计数略高于步数(因为我们没有使用任何衰减)。两者之间的差异是由于 ParallelEnv
创建了一个虚拟环境来初始化共享的 TensorDict
,该 TensorDict
用于从调度的环境中收集数据。这种微小的差异通常会在整个训练过程中被吸收。
print(
"update counts: ",
make_env.state_dict()["_extra_state"]["td"]["observation_count"],
)
env.close()
del env
脚本的总运行时间: (3 分钟 27.917 秒)
估计内存使用量: 2965 MB