Torchscript 支持¶
此示例说明了 torchvision transforms 在 Tensor 图像上的 torchscript 支持。
from pathlib import Path
import matplotlib.pyplot as plt
import torch
import torch.nn as nn
import torchvision.transforms as v1
from torchvision.io import decode_image
plt.rcParams["savefig.bbox"] = 'tight'
torch.manual_seed(1)
# If you're trying to run that on Colab, you can download the assets and the
# helpers from https://github.com/pytorch/vision/tree/main/gallery/
import sys
sys.path += ["../transforms"]
from helpers import plot
ASSETS_PATH = Path('../assets')
大多数 transforms 支持 torchscript。对于组合 transforms,我们使用 torch.nn.Sequential
而不是 Compose
dog1 = decode_image(str(ASSETS_PATH / 'dog1.jpg'))
dog2 = decode_image(str(ASSETS_PATH / 'dog2.jpg'))
transforms = torch.nn.Sequential(
v1.RandomCrop(224),
v1.RandomHorizontalFlip(p=0.3),
)
scripted_transforms = torch.jit.script(transforms)
plot([dog1, scripted_transforms(dog1), dog2, scripted_transforms(dog2)])
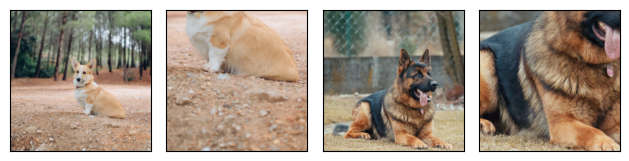
警告
上面我们使用了来自 torchvision.transforms
命名空间的 transforms,即 “v1” transforms。来自 torchvision.transforms.v2
命名空间的 v2 transforms 是在代码中使用 transforms 的推荐方式。
v2 transforms 也支持 torchscript,但是如果您对 v2 类 transform 调用 torch.jit.script()
,您实际上最终会得到其(脚本化的)v1 等效项。由于 v1 和 v2 之间的实现差异,这可能会导致脚本化执行和 eager 执行之间略有不同的结果。
如果您确实需要 v2 transforms 的 torchscript 支持,我们建议从 torchvision.transforms.v2.functional
命名空间脚本化 functionals 以避免意外。
下面我们展示如何结合图像转换和模型前向传递,同时使用 torch.jit.script
获取单个脚本化模块。
让我们定义一个 Predictor
模块,该模块转换输入 tensor,然后在上面应用 ImageNet 模型。
from torchvision.models import resnet18, ResNet18_Weights
class Predictor(nn.Module):
def __init__(self):
super().__init__()
weights = ResNet18_Weights.DEFAULT
self.resnet18 = resnet18(weights=weights, progress=False).eval()
self.transforms = weights.transforms(antialias=True)
def forward(self, x: torch.Tensor) -> torch.Tensor:
with torch.no_grad():
x = self.transforms(x)
y_pred = self.resnet18(x)
return y_pred.argmax(dim=1)
现在,让我们定义 Predictor
的脚本化和非脚本化实例,并将其应用于多个相同大小的 tensor 图像
device = "cuda" if torch.cuda.is_available() else "cpu"
predictor = Predictor().to(device)
scripted_predictor = torch.jit.script(predictor).to(device)
batch = torch.stack([dog1, dog2]).to(device)
res = predictor(batch)
res_scripted = scripted_predictor(batch)
Downloading: "https://download.pytorch.org/models/resnet18-f37072fd.pth" to /root/.cache/torch/hub/checkpoints/resnet18-f37072fd.pth
我们可以验证脚本化模型和非脚本化模型的预测是相同的
import json
with open(Path('../assets') / 'imagenet_class_index.json') as labels_file:
labels = json.load(labels_file)
for i, (pred, pred_scripted) in enumerate(zip(res, res_scripted)):
assert pred == pred_scripted
print(f"Prediction for Dog {i + 1}: {labels[str(pred.item())]}")
Prediction for Dog 1: ['n02113023', 'Pembroke']
Prediction for Dog 2: ['n02106662', 'German_shepherd']
由于模型是脚本化的,因此可以轻松地转储到磁盘并重新使用
import tempfile
with tempfile.NamedTemporaryFile() as f:
scripted_predictor.save(f.name)
dumped_scripted_predictor = torch.jit.load(f.name)
res_scripted_dumped = dumped_scripted_predictor(batch)
assert (res_scripted_dumped == res_scripted).all()
脚本的总运行时间: (0 分钟 1.428 秒)